Translate this page into:
Developing hypotheses for scientific research in musculoskeletal conditions: A guide for health-care professional
*Corresponding author: Adarsh Kumar Srivastav, School of Health Sciences, Chhatrapati Shahu Ji Maharaj University, Kanpur, Uttar Pradesh, India. adarshsrivastava13@gmail.com
-
Received: ,
Accepted: ,
How to cite this article: Rafat S, Srivastav AK. Developing hypotheses for scientific research in musculoskeletal conditions: A guide for health-care professional. J Musculoskelet Surg Res. 2024;8:189-97. doi: 10.25259/JMSR_117_2024
Abstract
The importance of hypothesis formulation in health-care research cannot be overstated. Hypotheses serve as a guiding framework for scientific inquiry. This narrative review highlights the characteristics, significance, and applications of hypotheses across musculoskeletal (MSK) research. Utilizing various databases, articles were scrutinized to delineate the role of hypotheses in research, education, and practice among MSK health-care professionals. The review underscores the pivotal role of hypotheses in advancing evidence-based practices and fostering critical thinking in MSK research.
Keywords
Evidence-based practice
Hypothesis
Qualitative research
Research design
Resource allocation
INTRODUCTION
Formulating hypotheses for any scientific research is significant for health-care professionals because it guides research, promotes evidence-based practice, enhances critical thinking, augments medical knowledge, improves resource allocation, and ensures ethical conduct.
When health professionals evaluate scientific reports about any intervention or experiment, it is essential to know whether reports are reliable and based on valid and appropriately conducted studies. For this purpose, some elements must be considered. The construction of a hypothesis and the experimental design to test the hypothesis are elementary components of the scientific method. Hypotheses are important for health-care professionals in different ways [Figure 1]. By constructing and testing hypotheses, health-care professionals enhance patient care, scientific understanding, and global health.
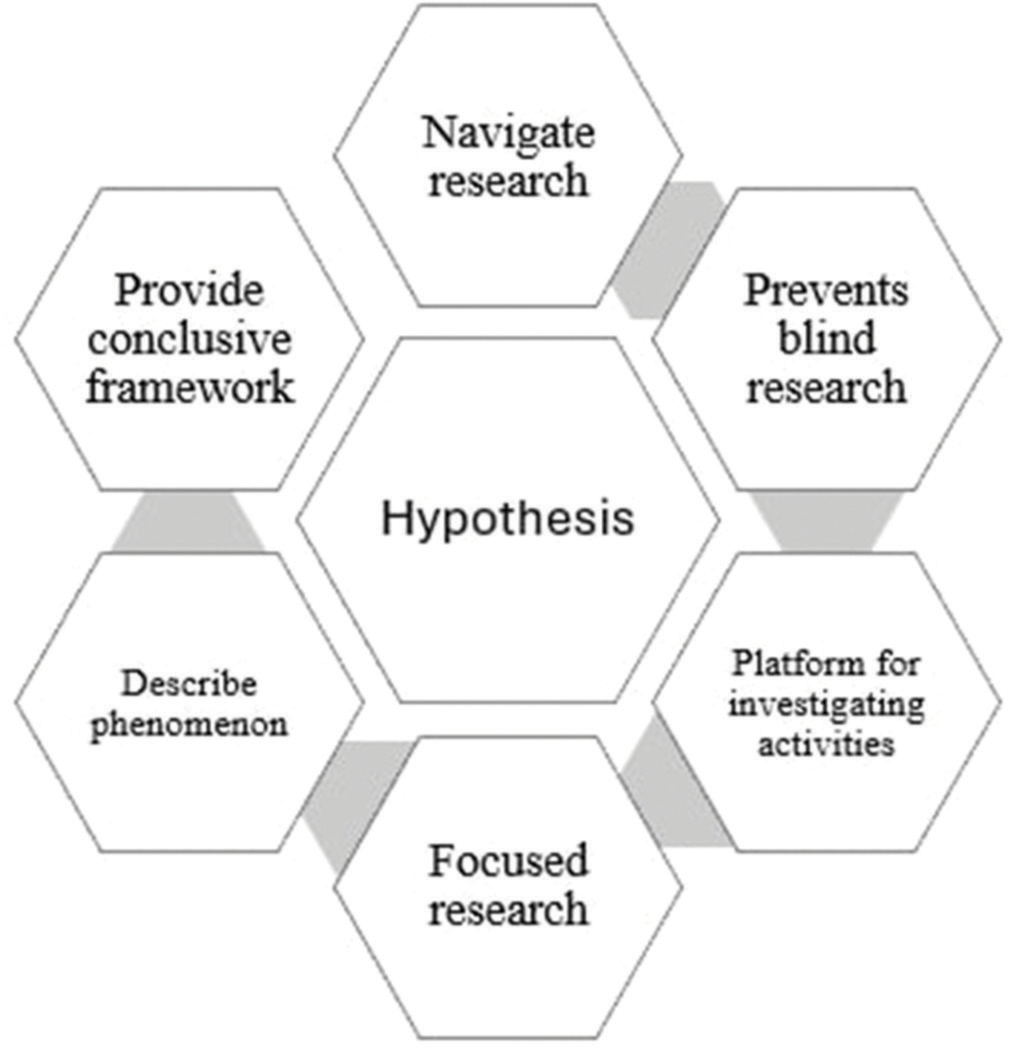
- Importance of hypothesis for health care professional.
The available literature on hypothesis development is broad,[1,2] but there is a paucity of resources that specifically target health-care professionals in musculoskeletal (MSK) care. This review aimed to bridge this gap by providing a comprehensive guide to hypothesis development for MSK health-care professionals.
This review aimed to highlight the significance of framing a clear and testable hypothesis grounded in existing literature as the research base. To test the hypothesis, a methodologically competent study must be designed, data must be gathered and analyzed, and reliable results must be confirmed. Interpreting these findings should reveal meaningful conclusions relevant to the research question and broader scientific knowledge.
WHAT IS HYPOTHESIS
The research study’s completion depends critically on the research hypothesis. Without the research hypothesis, we would not be able to discover all the essential components of the study method, and the conclusions’ applicability would be constrained.[3] A hypothesis is a tentative prediction or educated guess suggesting a possible explanation for a phenomenon or the relationship between dependent and independent variables. It serves as a refined and precise statement of the research problem, guiding the direction of experimental and exploratory studies.[4,5]
In clinical trials and research, a hypothesis is a provisional supposition accepted as highly reasonable based on known evidence. It directs inquiry and helps achieve conclusions by predicting relationships between variables, making research more focused and systematic.[6-8]
A good proposition must be established on a good research question. It should be testable, simple, specific, logical, clear and concise, falsifiable, parsimonious, refined, predictive, observable, and measurable.[3,9]
TYPES OF HYPOTHESES
Hypotheses can vary depending on the nature of the research and study design being conducted. Different study designs utilize various types of hypotheses. However, formulating a hypothesis is essential for studies that employ statistical significance tests to compare findings.[10] The different types of hypotheses are classified in Figure 2.
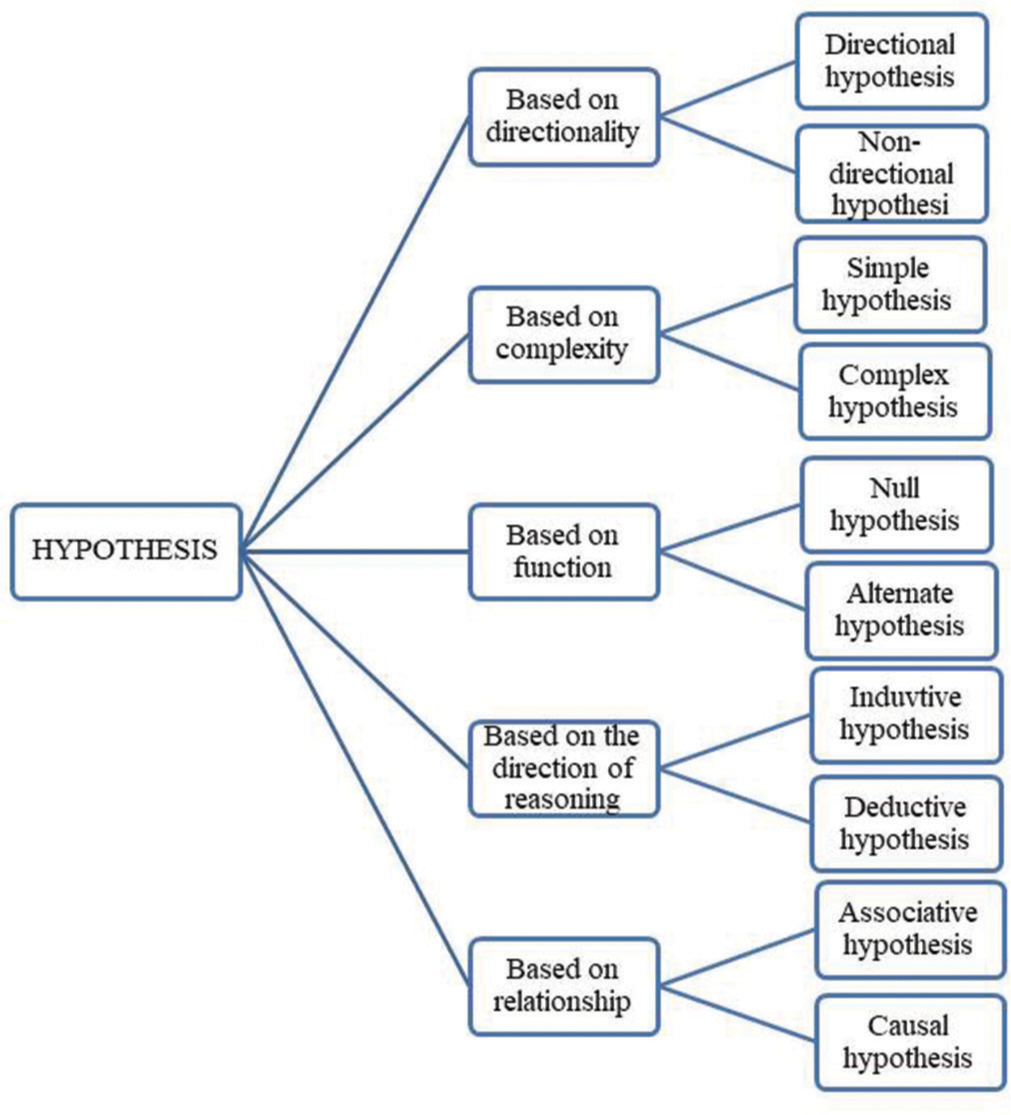
- Classification of hypothesis.
Based on directionality
Directional hypothesis
The directional hypothesis describes the association between variables in terms of a difference but also gives a direction to that difference. The directional hypothesis is also known as the unidirectional hypothesis. Different study designs develop directional hypotheses, which predict the relationship between one variable and another. They may be supported by solid theoretical justification or consistent earlier findings, particularly in experimental settings or meta-analyses. However, careful presentation is essential, recognizing that unexpected outcomes are possible. Remember that both directional and non-directional (predicting only a difference) hypotheses maintain value based on existing knowledge, even though the studies that generate them vary between fields.[11,12] For example, children with cerebral palsy who receive direct myofascial release technique in combination with functional training will have a significantly faster resolution of contracture, greater reduction of spasticity, and greater improvement in gross motor function when compared with children who receive functional training alone.
Non-directional hypotheses
Non-directional hypotheses, predicting a relationship without direction, frequently emerge when knowledge is constrained (new phenomenon, conflicting previous finding) or exploratory is key (exploratory studies, many variables). A non-directional hypothesis, also known as a bi-directional hypothesis, suggests that two variables change each other. It implies an equal relationship where both variables can affect each other. Even without guidance, they remain helpful since they can lead to unexpected findings and a deeper understanding, particularly in controversial or developing fields of study.[11,13] For example, there is an association between social media use and mental health, but the direction of the effect is uncertain. Brown et al. (2021)[14] conducted research comparing manual and exercise therapy’s effectiveness in treating shoulder pain. They hypothesized that there would be differences in the outcomes between the two therapies. Involving 150 patients randomly assigned to either manual or exercise therapy over 12 weeks, the study measured outcomes such as pain intensity, range of motion, and patient-reported functional status. The results indicated significant differences between the treatment groups, with varying directions and magnitudes across different measures. This non-directional hypothesis facilitated an unbiased assessment of both therapies’ effectiveness.
Based on complexity
Simple hypothesis
A simple hypothesis forecasts a relationship or difference between variables without specifying the direction and nature of the relationship. It consists of one independent variable and one dependent variable. It is commonly used in observational (cross-sectional and case–control) studies,[15] experimental (pre-test/post-test control group and pilot) studies,[16] and sometimes in case reports or descriptive studies; formulation of a simple hypothesis is needed for further exploration.[17] While simple hypotheses’ clarity and focused approach are advantageous, they are inappropriate for addressing complicated relationships or interactions between multiple variables. For example, to illustrate the application of simple hypotheses in MSK research, we can consider a few specific examples. In a study by O'Bryan et al. (2020), researchers hypothesized that a 12-week strength training program would reduce pain in patients with chronic low back pain (LBP).[18] The hypothesis was straightforward: Participating in the strength training program would lead to a decrease in pain levels. The study involved 100 participants who engaged in supervised strength training sessions; with pain levels measured using a standardized pain scale before and after the intervention. The results supported the hypothesis, showing a significant reduction in pain among participants.
Complex hypotheses
A complex hypothesis contains multiple variables and predicts more complex relationships between them. It may specify the influence of the interface among variables. The choice of study design for research using a complex hypothesis can be determined by various circumstances involving the definite nature of the hypothesis, the research questions being addressed, and the available resources. However, some common designs that are well-suitable for complex hypotheses include:
Experimental (randomized controlled trials [RCTs], factorial designs) studies,
Observational (cohort and case–control) studies, and
Mixed method studies combine qualitative and quantitative methods to collect data because they involve both subjective and objective factors.[16,19]
For example, several detailed examples can be explored to demonstrate the use of complex hypotheses in MSK research. In a study by Thompson et al. (2020), researchers proposed that combining strength training with dietary supplementation would improve muscle mass and functional performance in elderly patients with sarcopenia compared to either intervention alone.[20] This hypothesis suggested that the interaction between exercise and nutritional support would provide synergistic benefits. The study included 200 participants who were randomly assigned to one of four groups: Strength training, dietary supplementation, combined interventions, or a control group. Outcomes such as muscle mass, grip strength, and walking speed were measured before and after a 12-week intervention period. The results confirmed the hypothesis, showing that the combined intervention group experienced the most significant improvements across all measures.
Based on function
Null hypothesis
When there is no significant relationship or association between independent and dependent variables, the statistical hypothesis is called the null hypothesis (H0).[4] In hypothesis testing, it is the default position, and researchers try to invalidate it using statistical analysis. Here is a summary of the most common types of study design and how they use H0:[16,21]
Observational (cohort, case–control, and cross-sectional) studies,
Experimental RCT studies and
Systematic reviews and meta-analysis – They do not usually do H0 testing. However, it is a useful tool for determining the overall strength of the evidence in favor of or against a specific hypothesis.
For example, in a recent investigation by Garcia et al. (2021), researchers explored how various stretching techniques affect hamstring flexibility among athletes.[22] The H0 posited that there would be no discernible variance in hamstring flexibility enhancement between athletes engaging in static stretching versus those practicing dynamic stretching. The study encompassed 80 athletes, each randomly allocated to one of three groups: static stretching, dynamic stretching, or a control group. Evaluations of hamstring flexibility were conducted using the sit-and-reach test both before and after a four-week intervention phase. The outcomes demonstrated no statistically significant disparity in flexibility enhancement between the two stretching regimens, thereby corroborating the H0.
Alternate hypothesis
In contrast to the H0, the alternative hypothesis suggests that there is a significant association or difference between the variables. It is represented as H1 or Ha. Accepting the alternative hypothesis instead of the H0 implies that there is a difference between the groups or that the variables are connected.[23] The alternative hypothesis cannot be tested directly; it is accepted by rejection if the test of statistical significance rejects the H0. Although most studies do not generate alternate hypotheses directly, they can be developed with the help of a variety of research techniques. Various studies used alternative hypotheses, and these are: [24]
Observational studies
Descriptive studies – These studies do not test hypotheses. They can spot patterns and linkages in the data that point to possible alternative research hypotheses[25]
Correlational studies – These studies evaluate the connections between variables, but they do not prove cause and effect. Observing a connection between two variables can lead to different theories regarding possible causes.[26]
Literature reviews:
Systematic review – these reviews comprehensively analyze existing research on a particular topic and detect knowledge gaps. These gaps can act as starting points for alternate hypotheses to explore further. For example, a systematic review of individuals with knee osteoarthritis (OA) may encounter conflicting information about various exercise programs. This could prompt them to explore personalized exercise routines or investigate the effectiveness of specific exercises for their condition.[27]
Qualitative research (Interviews, focus groups)[28] exploratory research (pilot and feasibility studies)[19]
Case studies (rare or unique cases).[29]
For example, to demonstrate the application of alternate hypotheses in MSK research, consider the following scenarios. A recent study by Izquierdo et al. (2021) aimed to determine whether a novel resistance training method would yield superior gains in muscle mass compared to conventional weightlifting techniques.[30] The alternate hypothesis suggested that participants undergoing the new resistance training protocol would show significantly greater increases in muscle mass than those following traditional weightlifting methods. The study included 100 participants randomly allocated to either the experimental or control group. Muscle mass was assessed using dual-energy x-ray absorptiometry (DEXA) before and after a 12-week training period. The results revealed that individuals in the experimental group experienced notably higher gains in muscle mass than those in the control group, thus supporting the alternate hypothesis.
Based on the direction of reasoning
Inductive hypotheses
Using specific observations as a starting point, inductive reasoning generates new concepts and investigates poorly understood phenomena. For example, imagine viewing white swans and concluding that they are all white. This method, meanwhile, may lead to oversimplification.[24]
Many studies use inductive hypotheses for generalized conclusions and these are:
Exploratory studies
In these types of studies, the aim is to identify novel occurrences and provide a preliminary understanding of them. For instance, the inductive hypothesis that a new medication increases healing rates may be supported by the observation that multiple patients recover more quickly after using it.[31]
Grounded theory
In grounded studies, theories are generated directly from qualitative data through systematic theme and pattern analysis. For example, conducting interviews with participants regarding their experiences with the counseling programs regarding health may result in the inductive premise that health depends heavily on peer support.[32]
For example, a recent Garcia et al. (2020) investigation examined the connection between physical activity levels and bone mineral density (BMD)in postmenopausal women. Utilizing inductive reasoning, the researchers postulated that heightened physical activity levels would correlate with increased BMD in this demographic.[33] The study comprised 150 postmenopausal women whose physical activity levels were gauged through self-reported surveys, while BMD was measured through DEXA scans. The outcomes unveiled a positive correlation between physical activity and BMD, suggesting that regular physical activity may contribute to preserving bone health in postmenopausal women.
Deductive hypothesis
A deductive hypothesis is derived from theory and is designed to establish evidence that supports, expands, or contradicts properties of a given theory. Conversely, deductive hypotheses undermine accepted ideas.[5,31]
Studies using deductive hypothesis are as follows:
Hypothesis testing
Aims to validate or refuse hypotheses that have been derived from previous research. For instance, a scientist might put out the deductive idea that light bends around big objects based on the theory of relativity. After that, they would design experiments to verify this hypothesis.[34]
Confirmation bias research
It investigates the tendency of people to look for evidence to support their pre-existing ideas. A healthcare professional might, for instance, propose the deductive hypothesis that individuals who believe in conspiracy theories are more prone to believe in self-exercise than in evidence-based guidelines for chronic shoulder pain. Then, to verify this prediction, they conduct surveys and experiments.[31]
To demonstrate the application of deductive hypotheses in MSK research, consider these instances. In a study conducted by O'Bryan et al. (2021), researchers aimed to validate a deductive hypothesis suggesting that individuals with a consistent history of resistance training would display higher BMD than those without prior engagement in such activities.[18] The hypothesis was rooted in the understanding that resistance training, known for its capacity to stimulate bone growth, would enhance BMD over time. The study enrolled 200 participants, half with a documented history of regular resistance training, while the remainder had not participated in such exercises. The BMD was quantified using DEXA scans. The findings affirmed the deductive hypothesis, demonstrating notably elevated BMD levels among individuals with a history of resistance training compared to those without.
Associative hypothesis
An associative hypothesis suggests that a modification in one variable results in a change in another variable, but it does not determine which variable is causing the changes in the other. Interdependency between two variables is termed an associative hypothesis but cannot establish causality (cause-and-effect).[35] For example, there is an association between long-term sloughed sitting and an increased risk of neck pain.
Studies that use associative hypothesis are as follows:
Observational studies
Gather data through observation without active manipulation of variables. Examples: Surveys, case–control studies, and cohort studies. For example, improved cardiovascular health positively correlates with increased activity levels (Note: Other factors, such as food, may contribute to improved cardiovascular health; exercise is not the sole cause).[36]
In MSK research, associative hypotheses are vital in exploring potential relationships between variables. Lee and Patel (2021) investigated the association between physical activity levels and joint health in individuals with OA. The associative hypothesis proposed that higher levels of physical activity would be correlated with improved joint function and reduced pain in this population. The study involved 200 OA participants whose physical activity levels were assessed using activity trackers, while joint function and pain levels were measured through standardized assessments. The results revealed a significant association between higher physical activity levels, better joint function, and reduced pain, supporting the associative hypothesis.
Causal hypothesis
The causal hypothesis proposes a cause-and-effect interaction between variables. It indicates that manipulating one variable (independent) directly causes changes in another variable (dependent).[35] For example, not treating anterior cruciate ligament tears properly will cause OA.
Studies that use causal hypothesis are as follows:
Experimental studies
Actively change an independent variable and examine its effects on the dependent variable. Examples: RCTs and A/B testing (type of RCT experiment in which you run two different exercises simultaneously and see which performs better). For example, a study by Nguyen et al. (2020) aimed to establish a causal relationship between smoking and BMD in young adults.[37] The causal hypothesis suggested that smoking would lead to decreased BMD over time due to its detrimental effects on bone metabolism. The study involved 200 young adults, half smokers, and half non-smokers, with BMD measured using DEXA scans. The results showed a significant reduction in BMD among smokers compared to non-smokers, supporting the causal hypothesis.[36]
Various types of hypotheses and their corresponding study designs are summarized in Table 1.
Hypothesis type | Study design |
---|---|
Directional hypothesis | Quantitative studies (e.g., experiments, meta-analysis, surveys) |
Non-directional hypothesis | Exploratory studies, Quantitative studies (Experiments, surveys) |
Simple hypothesis | Observational (cross-sectional and case-control) studies, Experimental (pre-test/post-test control group and pilot) studies[16] and, sometimes, in a case report or descriptive studies |
Complex hypothesis | Experimental (RCTs, factorial designs) studies, Observational (cohort and case–control) studies, and mixed-method studies. |
Null hypothesis | Observational (cohort, case–control, and cross-sectional) studies, Experimental (RCTs) studies, Systematic reviews and meta-analysis. |
Alternate hypothesis | Observational (descriptive, correlational) studies, literature (systematic) reviews, and qualitative research (interviews), Exploratory research (pilot and feasibility studies), and case studies. |
Inductive hypothesis | Exploratory studies, Grounded theory. |
Deductive hypothesis | Hypothesis testing, confirmation bias research. |
Associative hypothesis | Observational studies (surveys, case–control studies, and cohort studies). |
Causal hypothesis | Experimental studies (RCTs, A/B testing). |
RCTs: Randomized control trials
HYPOTHESIS IN QUALITATIVE AND QUANTITATIVE STUDIES
In a quantitative study, a hypothesis is developed based on theory or information gathered from reading relevant literature. Before beginning a study, qualitative researchers typically do not formulate formal hypotheses.[5] On the other hand, a qualitative researcher might direct theories for the suggested study. Once a guiding hypothesis has been established, the qualitative researcher can operationalize it by formulating research questions that give the data-gathering process a clear direction. As a result of their investigation, qualitative researchers are likely to create new theories.[5]
STATISTICAL UNDERPINNINGS AND CONSIDERATIONS IN HYPOTHESIS TESTING
Hypothesis testing in MSK research involves inferring population characteristics based on sample data. The process starts with formulating an H0, which generally asserts no effect or no difference, and an alternative hypothesis, which posits the existence of an effect or a difference. The goal is to evaluate whether the observed data provide sufficient evidence to reject the H0 in favor of the alternative.[38]
Significance level (α)
The significance level, denoted by alpha (α), is the threshold probability for rejecting the H0, representing the risk of a Type I error (incorrectly rejecting a true H0). Common significance levels are 0.05, 0.01, and 0.10. For instance, an α of 0.05 means that there is a 5% chance of concluding that an effect exists when it actually does not.
P-values
P-value indicates the probability of obtaining results as extreme as those observed, assuming the H0 is true. If P-value is less than or equal to the chosen α, it suggests strong evidence against the H0, leading to its rejection. For example, with P = 0.03 and α = 0.05, we reject the H0, indicating statistical significance.
Statistical power and sample size
Statistical power is the probability of correctly rejecting a false H0 (avoiding a Type II error). Power is influenced by the sample size, effect size, and significance level. Conducting a power analysis during the study design phase helps determine the necessary sample size to detect a meaningful effect. Typically, a power of 0.80 (80%) is deemed sufficient, indicating an 80% chance of detecting an effect if it exists.
Effect size
Effect size measures the magnitude of the difference between groups or the strength of a relationship between variables, providing practical significance beyond mere statistical significance. Common effect size metrics include Cohen’s d for mean differences, Pearson’s r for correlations, and odds ratios for binary outcomes.
Confidence intervals (CIs)
CIs offer a range within which the true population parameter likely lies, with a certain confidence level (usually 95%). A 95% CI means that 95% of the calculated intervals would contain the true parameter if the study were repeated numerous times. CIs complement P-values by providing information about the estimate’s precision and uncertainty.
Controlling for confounding variables
Confounding variables are extraneous factors that can affect the study’s outcome, leading to misleading conclusions. Controlling for confounders is vital to isolate the effect of the independent variable on the dependent variable. Strategies include randomization, matching, stratification, and statistical adjustments such as multivariable regression analysis.
Types of statistical tests
The choice of statistical test depends on the study design, type of data, and variable distribution. Common tests in MSK research include:
t-tests: Compare means between two groups (e.g., independent t-test for two different groups, paired t-test for the same group at different times)
Analysis of variance: Compare means among three or more groups
Chi-square tests: Examine associations between categorical variables
Regression analysis: Explore relationships between one or more independent variables and a dependent variable (e.g., linear regression for continuous outcomes and logistic regression for binary outcomes).
Multiple testing and adjustment
Performing multiple statistical tests increases the risk of Type I errors. To address this, adjustments such as the Bonferroni correction can be applied, which involves dividing the significance level by the number of tests performed. Alternatively, methods like the false discovery rate control can balance the trade-off between detecting true effects and limiting false positives.[39]
Interpreting and reporting results
It is crucial to report statistical significance and the practical or clinical significance of the findings. This includes presenting effect sizes, CIs, and a clear interpretation of the results within the context of existing literature and clinical relevance.
For example, in an RCT comparing a new physical therapy protocol with standard care for reducing pain in patients with chronic LBP:
Null hypothesis (H0): No difference in pain reduction between the new physical therapy protocol and standard care
Alternative hypothesis (H1): The new physical therapy protocol reduces pain more than standard care
Statistical test: An independent t-test compares the mean pain scores between the two groups
Significance level: Set at α = 0.05
Power analysis: Conducted to ensure a sample size large enough to detect a clinically meaningful difference with 80% power
Effect size and CIs: Reported to indicate the treatment effect’s magnitude and precision.
COMMON PITFALLS AND CHALLENGES IN FORMULATING AND TESTING HYPOTHESES IN MSK RESEARCH
Researchers might unintentionally incorporate personal biases or preconceived notions into developing hypotheses, leading to skewed or invalid results. Encourage a comprehensive literature review and inclusion of diverse perspectives during hypothesis formulation to mitigate bias.[40]
Simplistic hypotheses might fail to capture the complexity of MSK conditions and the multifactorial nature of interventions. Develop detailed hypotheses considering multiple influencing factors, such as patient demographics, comorbidities, and treatment adherence.[41]
Ambiguities in defining key variables and outcomes can lead to inconsistent data collection and analysis, compromising study validity. Clearly define all variables and outcomes using standardized criteria to ensure consistency and reproducibility.
Inadequate sample sizes or low statistical power can result in the inability to detect true effects or produce false-positive results. Perform power analyses during the study design phase to determine the appropriate sample size needed to achieve sufficient statistical power.[42]
Failing to account for confounding variables can lead to misleading conclusions about the relationships between studied variables. Identify potential confounders during study design and use statistical methods to control for these variables in the analysis.
Flaws in study design, such as lack of randomization or control groups, can undermine the validity of hypothesis testing. Employ rigorous study designs, such as RCTs, and adhere to established methodological standards.
Variability in data collection procedures can introduce measurement errors and reduce the reliability of findings. Standardize data collection protocols and thoroughly train research staff to ensure uniformity and accuracy.
Ethical considerations may be overlooked, leading to potential harm to participants or ethical violations. Conduct thorough ethical reviews and obtain necessary approvals from the Institutional Review Boards before commencing the study.
Findings from narrowly defined populations may not apply to broader patient groups. To enhance the generalizability of results, design studies with diverse and representative samples.
Unique results from single studies may not be replicable, questioning the reliability of the findings. Encourage replication studies and meta-analyses to confirm findings and establish robust evidence.
STRATEGIES TO OVERCOME CHALLENGES
Collaborate with multidisciplinary teams to incorporate varied expertise and perspectives, enhancing the quality and scope of hypothesis testing[43]
Apply sophisticated statistical methods to analyze data and control for confounding variables, thereby improving the robustness of findings[44]
Conduct pilot studies to test and refine hypotheses and study designs before launching full-scale research projects[45]
Provide ongoing training for researchers and clinicians on the latest methodologies, ethical standards, and best practices in hypothesis formulation and testing
Encourage sharing of data, methodologies, and findings through open-access platforms to facilitate transparency and reproducibility in research.[46]
CONCLUSION
This review stresses the indispensable role of hypotheses in healthcare in general and MSK research in particular, advocating for their inclusion in every scientific endeavor. It offers practical insights into crafting hypotheses, encouraging readers to embrace the discipline’s inherent challenges and opportunities. It seeks to empower health-care professionals to engage in robust, innovative, and ethically sound research initiatives that contribute meaningfully to improving patient care and advancing scientific discovery.
AUTHORS’ CONTRIBUTIONS
SR and AKS designed this paper/letter for the editor, provided the data material, interpreted the data, wrote the initial and final manuscript, and reviewed the literature. SR and AKS have critically reviewed and approved the final draft and are responsible for the manuscript’s content and similarity index.
ETHICAL APPROVAL
The Institutional Review Board approval is not required.
DECLARATION OF PATIENT CONSENT
Patient’s consent was not required as there are no patients in this study.
USE OF ARTIFICIAL INTELLIGENCE (AI)-ASSISTED TECHNOLOGY FOR MANUSCRIPT PREPARATION
The author confirms that there was no use of artificial intelligence (AI)-assisted technology to assist in writing the manuscript.
CONFLICTS OF INTEREST
There are no conflicting relationships or activities.
FINANCIAL SUPPORT AND SPONSORSHIP
This study did not receive any specific grant from funding agencies in the public, commercial, or not-for-profit sectors.
References
- Hypothesis and hypothesis testing in the clinical trial. J Clin Psychiatry. 2001;62(Suppl 9):5-8. discussion 9-10
- [Google Scholar]
- The importance of defining the hypothesis in scientific research. Int J Educ Admin Policy Stud. 2012;4:170-6.
- [CrossRef] [Google Scholar]
- Designing studies of medical tests In: Designing clinical research. United States: Lippincott Williams and Wilkins; 2013. p. :183-205.
- [Google Scholar]
- Foundations of clinical research: Applications to practice Upper Saddle River, NJ: Pearson/Prentice Hall; 2009.
- [Google Scholar]
- Educational research: Competencies for analysis and applications United Kingdom: Pearson; 2012.
- [Google Scholar]
- Research methodology: Methods and techniques New Delhi: New Age International; 2004.
- [Google Scholar]
- Concepts of hypothesis testing and types of errors In: Dosage form design parameters. United States: Academic Press; 2018. p. :257-80.
- [CrossRef] [Google Scholar]
- Research methodology and biostatistics-e-book: A comprehensive guide for health care professionals New York: Elsevier Health Sciences; 2016.
- [Google Scholar]
- The meaning of “significance” for different types of research [translated and annotated by eric-jan wagenmakers, denny borsboom, josine verhagen, rogier kievit, marjan bakker, angelique cramer, dora matzke, don mellenbergh, and han lj van der maas] Acta Psychol. 2014;148:188-94.
- [CrossRef] [PubMed] [Google Scholar]
- Is two-tailed testing for directional research hypotheses tests legitimate? J Bus Res. 2013;66:1261-6.
- [CrossRef] [Google Scholar]
- A practical guide to writing quantitative and qualitative research questions and hypotheses in scholarly articles. J Korean Med Sci. 2022;37:e121.
- [CrossRef] [PubMed] [Google Scholar]
- Inferring processes from spatial patterns: The role of directional and non-directional forces in shaping fish larvae distribution in a freshwater lake system. PLoS One. 2012;7:e50239.
- [CrossRef] [PubMed] [Google Scholar]
- A scoping review of chronic pain in emerging adults. Pain Rep. 2021;6:e920.
- [CrossRef] [PubMed] [Google Scholar]
- A concise introduction to mixed methods research United States: SAGE Publications; 2021.
- [Google Scholar]
- Student perspectives on learning research methods in the social sciences. Teach Higher Educ. 2019;25:797-811.
- [CrossRef] [Google Scholar]
- Progressive resistance training for concomitant increases in muscle strength and bone mineral density in older adults: A systematic review and meta-analysis. Sports Med. 2022;52:1939-60.
- [CrossRef] [PubMed] [Google Scholar]
- Nursing research: Generating and assessing evidence for nursing practice United States: Lippincott Williams and Wilkins; 2008.
- [Google Scholar]
- The effectiveness of two methods of prescribing load on maximal strength development: A systematic review. Sports Med. 2020;50:919-38.
- [CrossRef] [Google Scholar]
- The art of the null hypothesis-considerations for study design and scientific reporting. J Cardiothorac Vasc Anesth. 2023;37:867-9.
- [CrossRef] [PubMed] [Google Scholar]
- Analysis of bone impairment by 3D DXA hip measures in patients with primary hyperparathyroidism: A pilot study. J Clin Endocrinol Metab. 2020;105:175-84.
- [CrossRef] [PubMed] [Google Scholar]
- Developing the research hypothesis. J Invest Surg. 2011;24:191-4.
- [CrossRef] [PubMed] [Google Scholar]
- Formulating hypotheses for different study designs. J Korean Med Sci. 2021;36:e338.
- [CrossRef] [PubMed] [Google Scholar]
- Descriptive versus explanatory hypotheses in evolutionary research: A potentially concerning bias exemplified by research into the evolution of social organisations in carnivores. Ethol Ecol Evol. 2012;24:97-103.
- [CrossRef] [Google Scholar]
- Correlational data, causal hypotheses, and validity. J Gen Philos Sci. 2011;42:85-107.
- [CrossRef] [Google Scholar]
- The association of physical deconditioning and chronic low back pain: A hypothesis-oriented systematic review. Disabil Rehabil. 2006;28:673-93.
- [CrossRef] [PubMed] [Google Scholar]
- Using the terms “hypothesis” and “variable” for qualitative work: A critical reflection. J Marriage Fam. 2012;74:671-7.
- [CrossRef] [Google Scholar]
- Case studies: Types, designs, and logics of inference. Conflict Manag Peace Sci. 2008;25:1-8.
- [CrossRef] [Google Scholar]
- International exercise recommendations in older adults (ICFSR): Expert consensus guidelines. J Nutr Health Aging. 2021;25:824-53.
- [CrossRef] [PubMed] [Google Scholar]
- The potential of working hypotheses for deductive exploratory research. Qual Quant. 2021;55:1703-25.
- [CrossRef] [PubMed] [Google Scholar]
- Deductive qualitative analysis and grounded theory: Sensitizing concepts and hypothesis-testing In: The SAGE handbook of current developments in grounded theory. United States: Sage; 2019. p. :107-22.
- [CrossRef] [Google Scholar]
- Analysis of bone impairment by 3D DXA hip measures in patients with primary hyperparathyroidism: A pilot study. J Clin Endocrinol Metab. 2020;105:175-84.
- [CrossRef] [PubMed] [Google Scholar]
- Hypothesis testing and model. In: Markov chain Monte Carlo in practice. Vol 10. London: Chapman and Hall; 1995. p. :163-85.
- [Google Scholar]
- Causal and associative hypotheses in psychology: Examples from eyewitness testimony research. Psychol Public Policy Law. 2006;12:190.
- [CrossRef] [Google Scholar]
- Testing one hypothesis twice in observational studies. Biometrika. 2012;99:763-74.
- [CrossRef] [Google Scholar]
- Crowding-out effect of tobacco expenditure in Vietnam. Tob Control. 2020;29:s326-30.
- [CrossRef] [PubMed] [Google Scholar]
- Understanding statistical hypothesis testing: The logic of statistical inference. Mach Learn Knowl Extr. 2019;1:945-62.
- [CrossRef] [Google Scholar]
- Multiple hypothesis testing and Bonferroni's correction. BMJ. 2014;349:g6284.
- [CrossRef] [PubMed] [Google Scholar]
- Biased hypothesis formation from projection pursuit. arXiv:220100889. [arXiv preprint]
- [Google Scholar]
- The evolution of virulence in parasites and pathogens: Reconciliation between two competing hypotheses. J Theor Biol. 1994;169:253-65.
- [CrossRef] [PubMed] [Google Scholar]
- Significance, errors, power, and sample size: The blocking and tackling of statistics. Anesth Analg. 2018;126:691-8.
- [CrossRef] [PubMed] [Google Scholar]
- The benefits of multidisciplinary collaboration in scientific research. J Interdiscip Stud. 2018;45:123-36.
- [Google Scholar]
- Advanced statistical techniques for analyzing complex data sets. J Appl Stat. 2020;37:567-81.
- [Google Scholar]
- The importance of pilot studies in refining research methodologies. Res Des Methods. 2019;12:245-57.
- [Google Scholar]
- The impact of data sharing on research transparency and reproducibility. J Open Sci. 2021;5:210-25.
- [Google Scholar]